The XLSTAT statistical analysis add-in offers a wide variety of functions to enhance the analytical capabilities of Microsoft Excel, making it the ideal tool for your everyday data analysis and statistics requirements. The XLSTAT-Premium solution XLSTAT-Premium is an advanced yet affordably priced statistical solution that includes all of the 200+ XLSTAT features currently available. In XLSTAT-Premium we have included it all so you’ll have access to any method, any time. Prepare data, visualize, explore, analyze, take decisions, predict.
XLSTAT is a complete data analysis and statistics add-in for Excel. It includes regression (linear, logistic, nonlinear), multivariate data analysis, parametric tests, non parametric tests, ANOVA, ANCOVA, forecasting methods.
XLSTAT 365 is a user-friendly cloud-based application for statistics and data analysis that works as an add-on to Google Sheets. With XLSTAT 365 enjoy access to all mainstream statistical features anytime, anywhere from your PC, MAC, Tablet or Smartphone. XLSTAT 365 makes data analysis easier than ever as it operates seamlessly with Google Sheets. The XLSTAT trial is followed by a complimentary lifetime limited edition. Perform risk analysis in a vast range of fields and get answers to a huge range of questions using this intuitive simulation add-on for Microsoft Excel. XLSTAT has the module for performing the same, but I dont have access to the software as trial version is expired. I also need help arranging the data and selecting the variables.
XLSTAT is a complete analysis and statistics add-in for Excel. It has been developed since 1993.XLSTAT includes more than 240 features in general or field-specific solutions.
The use of Excel as an interface makes XLSTAT a user-friendly and highly efficient statistical and multivariate data analysis package.
It includes regression (linear, logistic, nonlinear), multivariate data analysis (Principal Component Analysis, Discriminant Analysis, Correspondence Analysis, Multidimensional Scaling, Agglomerative Hierarchical Clustering, K-means, K-Nearest Neighbors, Decision trees), correlation tests, parametric tests, non parametric tests, ANOVA, ANCOVA, mixed models and much more.
Xlstat Online
Add Xlstat Excel
Field-specific solutions allow for advanced multivariate analysis (RDA, CCA, MFA), Preference Mapping and other sensometrics tools, Statistical Process Control, Simulations, Time series analysis, Dose response effects, Survival models, Conjoint analysis, PLS modelling, Structural Equation Modelling, OMICS data analysis.. Optional modules include 3D Visualization and Latent Class models.The XLSTAT statistical analysis software is compatible with all Excel versions from 2003 to 2016. A Mac version is also available on the XLSTAT website, and works on Excel 2011 & 2016.
Version 2021.2: All XLSTAT solutions except Basic: DBSCAN machine learning algorithm.XLSTAT Sensory and Premium: Generalized Bradley-Terry model; the Balloon plot.XLSTAT Quality and Premium: Pareto Chart.XLSTAT Quality, Life Sciences and Premium: Mixture Designs.
Version 2021: * Available in all XLSTAT solutions: Discriminant Analysis* Available in all XLSTAT solutions except Basic: One-class Support Vector Machine Support Vector Machine Principal Component Regression* Available in XLSTAT Life Sciences, Quality & Premium: Response Surface Designs
Version 2020.5: New features, advanced options & improvements:Support Vector RegressionClustatisScreening DesignsK Nearest Neighbors (KNN)Naive Bayes Classification
Version 2020.3.1: New features, advanced options & improvements: Contingency Tables Pairwise Deletion Option Grouped and stacked bar charts Bar Chart Race Nonlinear Regression Descriptive Statistics Handy generic Options Bayesian networks (available in Life Sciences, Marketing and Premium Editions)
Version 2020.3: Available in all XLSTAT solutions:* Bar Chart Race* Nonlinear Regression* Descriptive Statistics* Contingency Tables* Handy generic XLSTAT OptionsAvailable in XLSTAT Life Sciences, Marketing and Premium:* Bayesian networks
Version 2019.4.1.0: Demšar Significance Diagram (Critical Differences plot)Latent Semantic AnalysisCustomer Long-term valueTaguchi experimental designsDesign of sensory discrimination tests
Version 2019.3.1: Matrix operator to perform operations on matrices: multiplication, addition, subtraction, inversion, transposition.Cochran Q test - the Critical difference (Sheskin) method for pairwise comparisons.Ability to display a proportions chart.New estimation method - Passing Bablok regression.
Version 2019.2.1: Data analysis on Excel filtered data.Probability Calculator.Nonlinear Regression.Stepwise Cox Regression.A new feature for analyzing temporal check-all-that-apply (TCATA) data.A new feature for processing CATA data.
Principles of Principal Component Analysis
Principal Component Analysis (PCA) is one of the most frequently used multivariate data analysis.
Principal Component Analysis can be considered as a projection method which projects observations from a p-dimensional space with p variables to a k-dimensional space (where k < p) so as to conserve the maximum amount of information (information is measured here through the total variance of the scatter plots) from the initial dimensions. Installing visual studio code on raspberry pi 4. If the information associated with the first 2 or 3 axes represents a sufficient percentage of the total variability of the scatter plot, the observations will be able to be represented on a 2- 3-dimensional chart, thus making interpretation much easier.
Use of Principal Component Analysis
There are several uses for Principal Component Analysis, including:
- The study and visualization of the correlations between variables to hopefully be able to limit the number of variables to be measured afterwards;
- Obtaining non-correlated factors which are linear combinations of the initial variables so as to use these factors in modeling methods such as linear regression, logistic regression or discriminant analysis.
- Visualizing observations in a 2- or 3-dimensional space in order to identify uniform or atypical groups of observations.
Principal Component Analysis input data
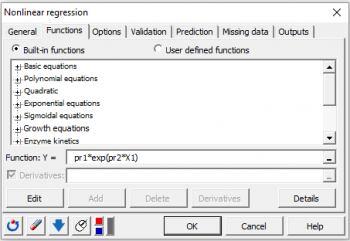
XLSTAT offers several possibilities for the matrix to be used in the Principal Component Analysis algotithm:
- Pearson (n) and (n-1)
- Covariance (n) and (n-1)
- Spearman
- Kendall
- Polychoric
Rotation for Principal Component Analysis
Rotations can be applied on the factors. Several methods are available including Varimax, Quartimax, Equamax, Parsimax, Quartimin and Oblimin and Promax.
Results for Principal Component Analysis in XLSTAT
Correlation/Covariance matrix
This table shows the data to be used afterwards in the calculations. The type of correlation depends on the option chosen in the 'General' tab in the dialog box. For correlations, significant correlations are displayed in bold.
Bartlett's sphericity test
The results of the Bartlett sphericity test are displayed. They are used to confirm or reject the hypothesis according to which the variables do not have significant correlation.
Eigenvalues

The eigenvalues and corresponding chart (scree plot) are displayed. The number of eigenvalues is equal to the number of non-null eigenvalues.
Factor loadings and correlations
XLSTAT displays the factor loadings in the new space, then the correlations between the initial variables and the components in the new space. The correlations are equal to the factor loadings in a normalized PCA (on the correlation matrix).
If supplementary variables have been selected, the corresponding coordinates and correlations are displayed at the end of the table.
Contributions
Contributions are an interpretation aid. The variables which had the highest influence in building the axes are those whose contributions are highest.
Squared cosines for the variables
As in other factor methods, squared cosine analysis is used to avoid interpretation errors due to projection effects. If the squared cosines associated with the axes used on a chart are low, the position of the observation or the variable in question should not be interpreted.
Factor scores
Xlstat Download
The factor scores in the new space are then displayed. If supplementary data have been selected, these are displayed at the end of the table.
Contributions
The contribution table shows the contributions of the observations in building the principal components.
Squared cosines for the obsrevations
The squared cosines table displays the squared cosines between the observation vectors and the factor axes.
Results with rotations
Where a rotation has been requested, the results of the rotation are displayed with the rotation matrix first applied to the factor loadings. This is followed by the modified variability percentages associated with each of the axes involved in the rotation. The coordinates, contributions and cosines of the variables and observations after rotation are displayed in the following tables.
XLSTAT charts for Principal Component Analysis
Xlstat Add In
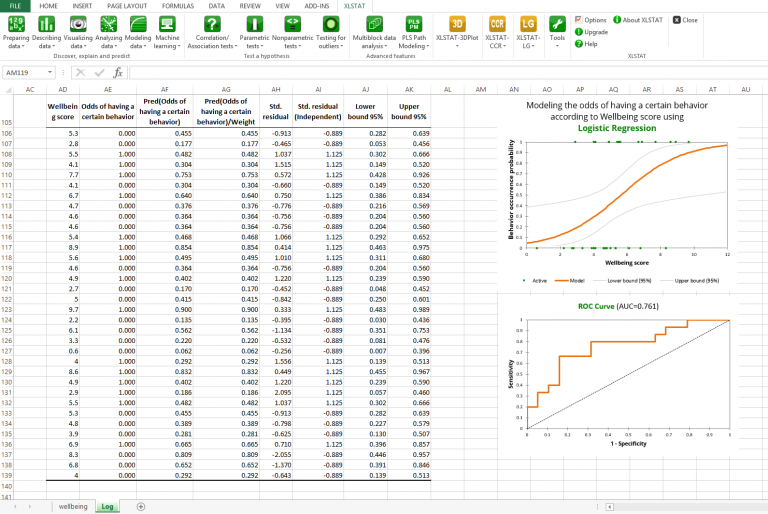
Correlations charts
These charts show the correlations between the components and initial variables.
Ios friday night funkin. You can also display the initial variables in the form of vectors.
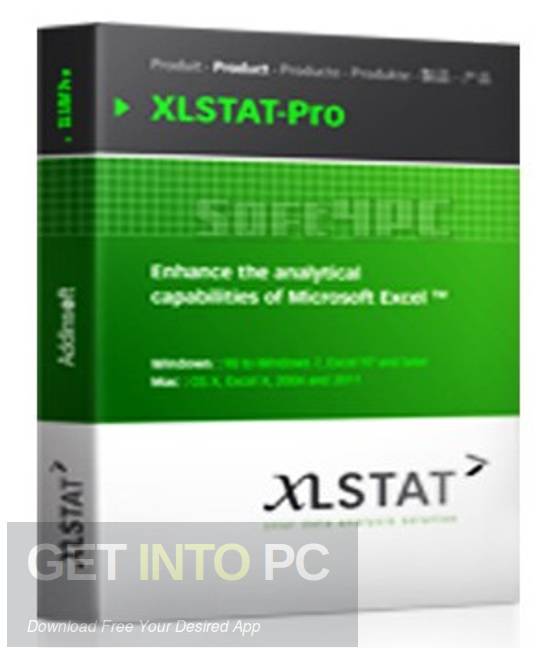
Observations charts:
The obsrevations charts represent the observations in the new space.
Biplots
The biplots represent the observations and variables simultaneously in the new space.
Here as welle the initial variables can be plotted in the form of vectors.
There are different types of biplots:
Xlstat Calculator
- Correlation biplot
- Distance biplot
- Symmetric biplot
- Coefficient: Choose the coefficient whose square root is to be multiplied by the coordinates of the variables. This coefficient lets you to adjust the position of the variable points in the biplot in order to make it more readable. If set to other than 1, the length of the variable vectors can no longer be interpreted as standard deviation (correlation biplot) or contribution (distance biplot).
This analysis is available in the XLStat-Basic addin for Microsoft Excel™
